When
In April 2022, the NC CASC celebrated Earth Week by hosting Climate Solutions Days, an à la carte offering of presentations, workshops and trainings scheduled throughout the week where scientists and practitioners shared insights on climate change impacts from findings in the North Central region. Themes woven into panel presentations, working groups, listening sessions, trainings and tools included:
NC CASC March/April 2022 Newsletter Now Available Online
When
NC CASC Partners Co-Author Paper: "Challenges to Forest Restoration in an Era of Unprecedented Climate and Wildfire Activity in Rocky Mountain Subalpine Forests"
NC CASC consortium partners, Phil Higuera, University of Montana, and Shelley Crausbay, Conservation Science Partners (CSP), are co-authors on a new paper, "Challenges to forest restoration in an era of unprecedented climate and wildfire activity in Rocky Mountain sub
GPTWA Seeks Applicants for Tribal Climate Resilience Liaison II Position
The Great Plains Tribal Water Alliance is hiring for a full-time Tribal Climate Resilience Liaison. The Liaison will connect climate change resources, tools, and information to tribal resource managers and partners to support resiliency-building efforts across the North Central region (MT, WY, CO, ND, SD, NE, KS). For more information about the position, including how to apply, click here.
R-Shiny App to Project Daily Snowfall and Rainfall
The NC CASC Climate Science Support Platform has developed a new R-Shiny app to quantify daily snowfall and rainfall quantities from total daily precipitation data in both observations (gridMET) and future projections (MACAv2-METDATA). Often the observed and projected precipitation data do not distinguish between snowfall and rainfall. Based on our best current understanding, this new application uses relationship between the phase of precipitation and the temperature and relative humidity metrics to quantify proportion of daily precipitation falling as rainfall or snowfall.
Dr. Christy Miller-Hesed to Co-facilitate "Climate Change and Climate Adaptation Training for Grasslands Conservation Practitioners"
North American grasslands are a regional priority of the U.S. Fish and Wildlife Service (FWS). The South Central CASC, in partnership with the US FWS Science Applications Program, the Northwest CASC, and the North Central CASC, will be implementing a training series for grasslands conservation practitioners starting in May 2022. Through our training series, we will introduce practitioners to the science of climate change, explore the impacts, and discuss adaptation options available.
ESA Announces Emerging Indigenous Scholar Leadership Award
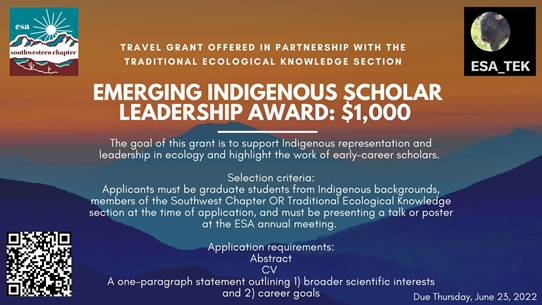
Contact Us
Want to see more? Do you have feedback? Was this site helpful? Send us an email!